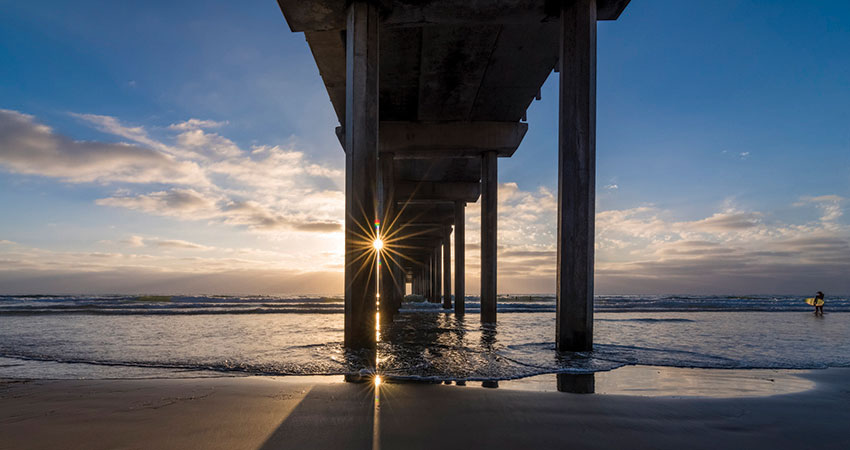
Talk Abstract: TBA
The climate system involves a variety of nonlinearly interacting physical processes spanning a broad range of spatial and temporal scales. To make simulations of the climate system computationally tractable, processes with scales smaller than the typical grid size of climate models have to be parameterized. Recently, there has been substantial interest (and progress) in using deep learning techniques to develop data-driven subgrid-scale (SGS) parameterizations for a number of key processes in the atmosphere, ocean, and other components of the climate system. In this talk, I will use 2D turbulence as a test case and discuss some of the recent progress as well as challenges and potential solutions in developing such data-driven SGS parameterizations. In particular, I will discuss issues related to stability (through connections to capturing backscattering), physics-constrained learning in the small-data regime, extrapolation (e.g., to higher forcing or Reynolds number) using transfer learning, and interpretation of what the deep neural networks have learned. I will further discuss how these advances will be used in a project aimed at developing SGS parameterizations for atmospheric gravity waves. I will also briefly mention some of our work on data-driven weather forecasting and integrating deep learning with data assimilation. Finally, I will say a few words about our work on large-scale atmospheric dynamics focused on blocking events and annular modes.
All seminars this quarter will be in a hybrid format. Recording available upon request.